Here at BrainAccess.ai, recently we trained a ‘fast’ P300 classifier and were impressed with the results. The new algorithm will be integrated into our eeg software in the near future.
P300 potential can be observed in EEG recordings when a stimulus of interest or an odd stimulus is shown to a person. The P300 paradigm can be employed within BCI system technologies to allow users to choose options on the screen or in studying the attention of people to different visual material. It can even be used to detect if a particular information is known by a person, in essence acting as a lie detector.
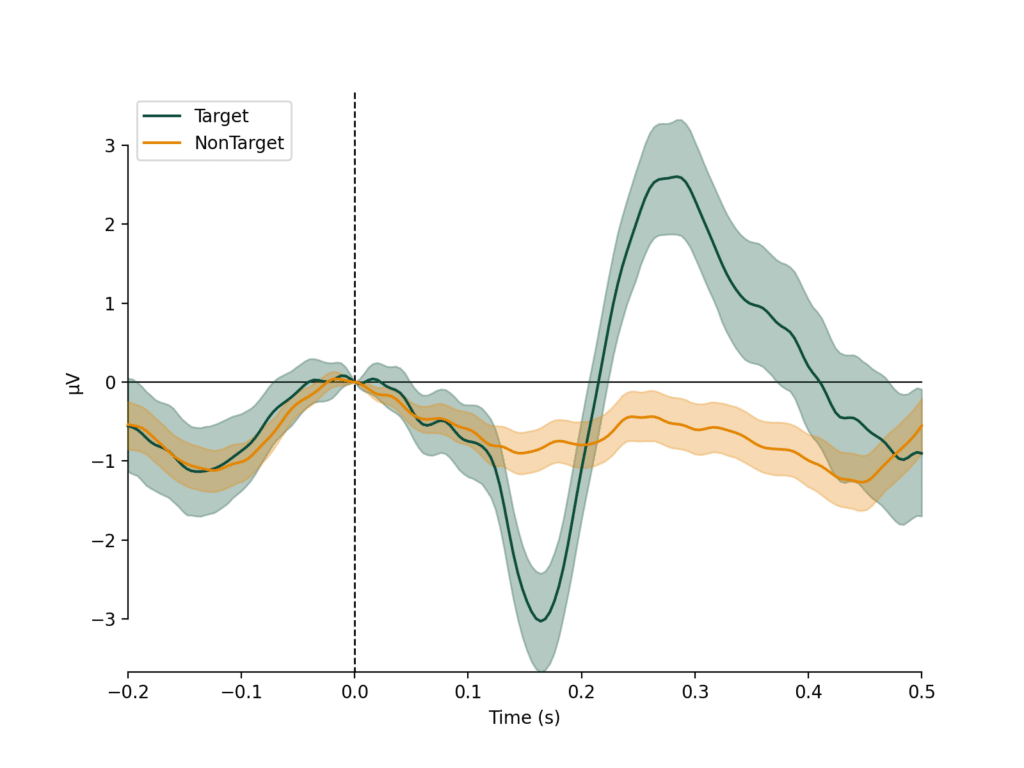
Detecting P300 potential in EEG waveforms is not an easy task as its amplitude is relatively small and is typically buried in a noisy signal. Many repetitions are usually made to recover and identify P300 potential in EEG signals. Our newly trained classifier can work with only 2 EEG electrodes at the occipital region (referenced at FPz) and require only 3 repetitions. This enables using the P300 paradigm with EEG headband systems that are cheaper and easier to set up than full EEG cap systems.
In addition to this, the detection algorithm is called ‘fast’ P300 classifier, as the time between two subsequent stimuli is 215 ms only. Conventional setups work with periods of more than 300 ms as the P300 potential is observed only after 300 ms after the stimulus. Hence, the ‘fast’ P300 classifier allows developing faster BCI systems.
Have a look at this video where the new classifier model is tested with a soon to be released BrainAccess HALO headband.